0. 前言
这篇论文是旷视科技 Video Team 做的。
这篇论文主要介绍 re-id 代码中的各种 trick 的作用。
1. Introduction
作者总结了各种 trick 并与 ECCV2018 CVPR2018 的论文做了比较,实验证明,只需要通过各种 trick 的组合,就比提出的算法高很多。
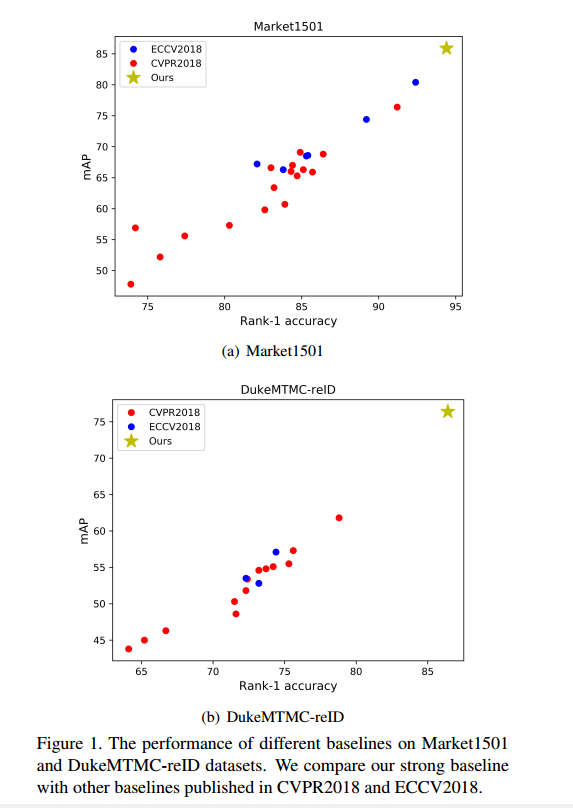
2. Standard Baseline
- ResNet50 为基础网络,修改最后一个 fc 层的输出维度为行人类别 $N$.
- 随机取 $P$ 个人, 每个人 $K$ 张图片,所以每个 batch $B=P\times K$,作者设置 $P=16, K=4$.
- 图片 resize 成 256x128,并添加10个0元素的 pad,然后随机 crop 成 256x128.
- 图片随机水平翻转概率设置为0.5.
- 图片的像素值转化为 [0,1],并且 normalize: mean=0.485, 0.456, 0.406, std=0.229, 0.224, 0.225.
- 模型提取特征记为 $f$,ID 的预测值为 $p$.
- ReID features $f$ 用于计算 triplet loss,ID prediction logits $p$ 用于计算交叉熵,margin $m=0.3$
- 优化器: Adam: lr=0.00035, epoch=120, 第40和第70个 epoch 乘 0.1.
3. Training Tricks
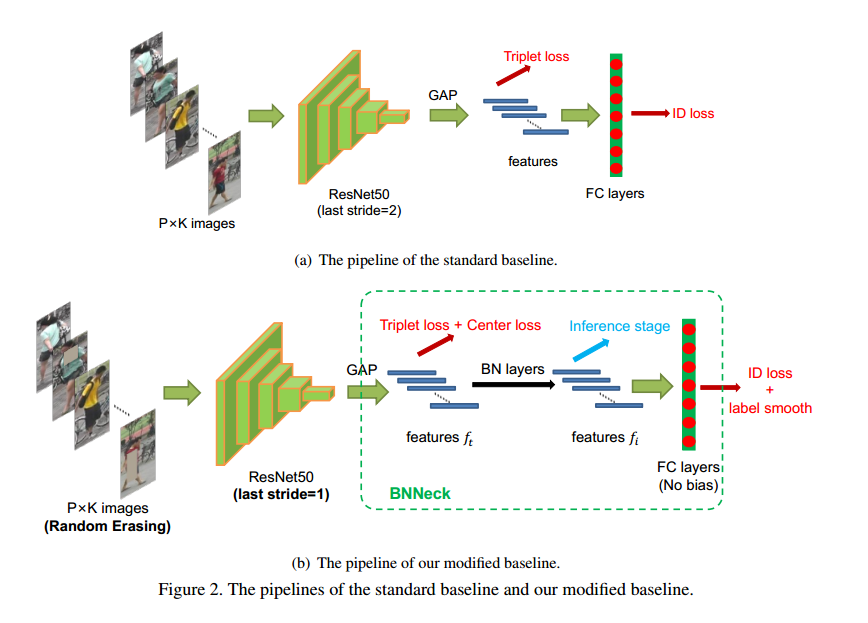
3.1 Warmup Learning Rate
Question: 前10个epoch是不是写错了,应该是-4,不是-5
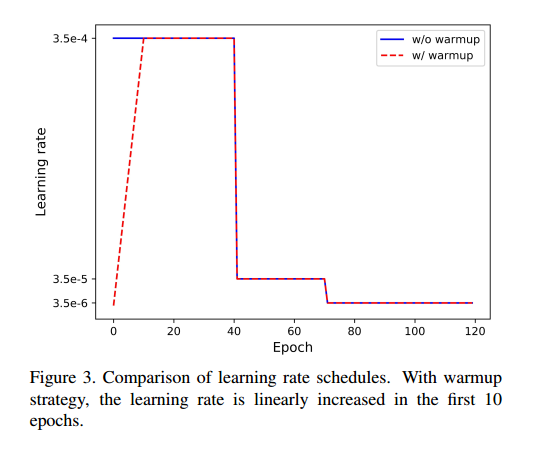
3.2 Random Erasing Augmentation
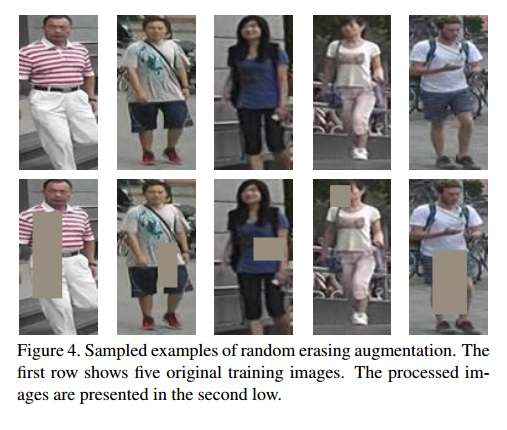
- probability: $p_e=0.5$
- rectangle region $I_e: S_e=W_e\times H_e$, $0.02<S_e<0.4$
- area ratio: $r_1< r_e=\frac{S_e}{S} < r_2$, $r_1=0.3, r_2=3.33$
3.3 Label Smoothing
$\epsilon=0.1$
3.4 Last Stride
- stride=2: 256x128->8x4
- stride=1: 256x128->16x8
3.5 BNNeck
- ID loss: 更偏向于 cosine distance
- triplet loss: 更偏向于 Euclidean distance
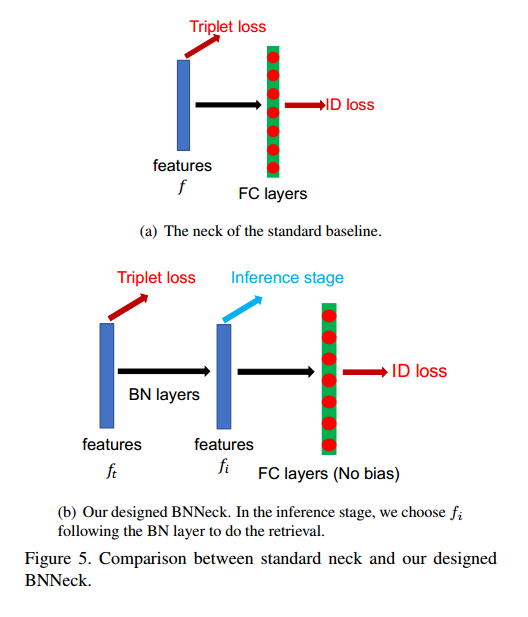
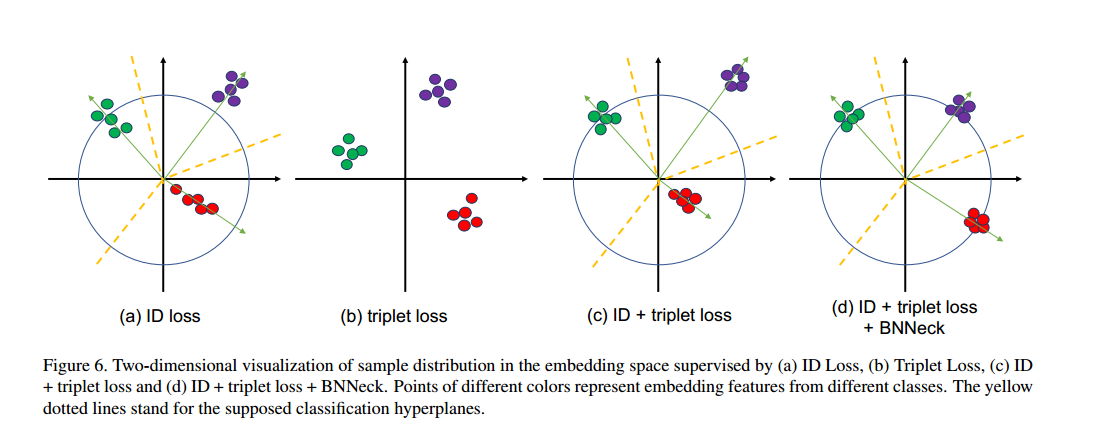
3.6 Center Loss
三元组损失只能使一个 batch 内的正负样本的值相差比较大,却不能考虑全局的正负样本值。
Triplet loss:
Center loss:
其中 $yj$ 是第 j 张图片的label,$c{yj}$ 表示第 $y_j$ 类的特征的中心,$f{t_j}$ 表示提取的特征 $f_t$.
Overall:
$\beta=0.0005$
4. Experimental Results
作者一共做了两组实验,一组是在 source damain 上的,一组是在 cross domain 上的。
4.1 Influences of Each Trich (Same domain)
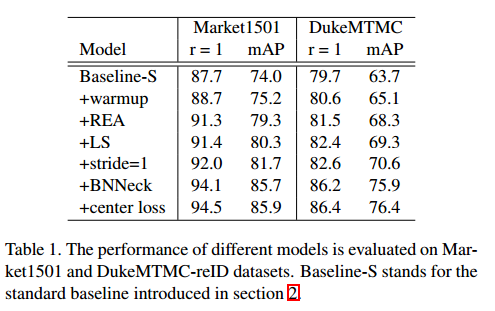
这些应该是在前面各种 trick 已经有的情况再加一个得到的结果。
4.2 Analysis of BNNeck
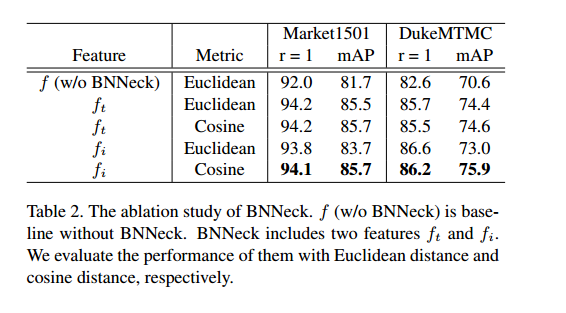
我觉得这个表格说明了 bn 层是用的,但是在测试的时候取 $f_t$ 还是 $f_i$ ,用 cosine 还是 Euclidean 是无所谓的。
4.3 Influences of Each Trick (Cross domain)
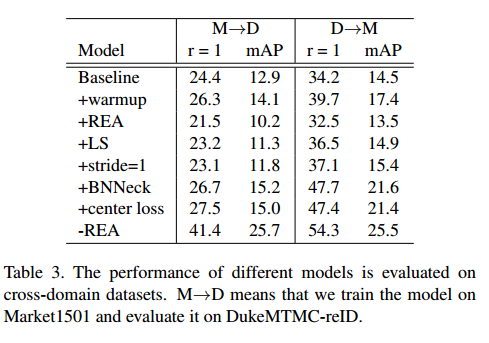
warmup and label smoothing 更有用一些,stride=1, center loss 没啥用,REA 有负作用。
5. Supplementary Experiments
5.1 Influences of the Number of Batch Size
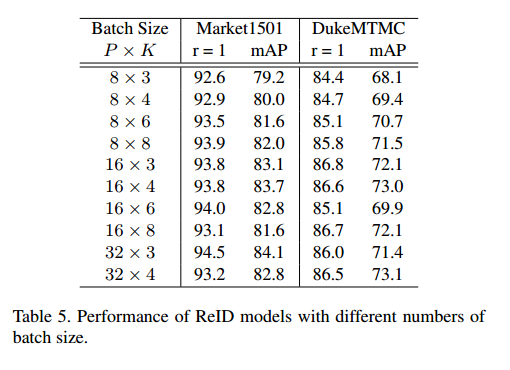
差别也就在2个点足有,不是特别大,但是更大的 batch 是更有用的。
5.2 Influences of Image Size
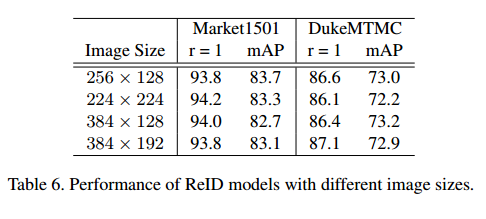
也差不太多