1. 前言
CVPR2019 看到好几篇都使用了 memory 的结构来进行 person re-id,所以对这几篇论文总结一下。具体的论文分析在我之前的博客已经有,这里主要介绍这几篇论文对 memory 的使用方法和效果。
- OIM: CVPR2017_Joint Detection and Identification Feature Learning for Person Search
- DIMN: CVPR2019_Generalizable Person Re-identification by Domain-Invariant Mapping Network
- MAR: CVPR2019 Unsupervised Person Re-identification by Soft Multilabel Learning
- ECN: CVPR2019_Invariance Matters Exemplar Memory for Domain Adaptive Person Re-identification
2. memory 的建立
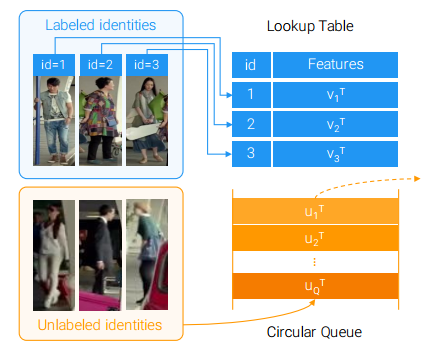
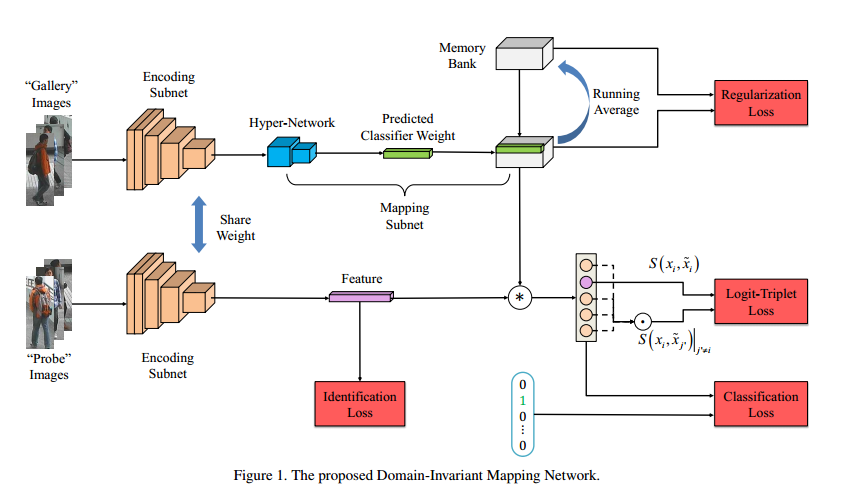
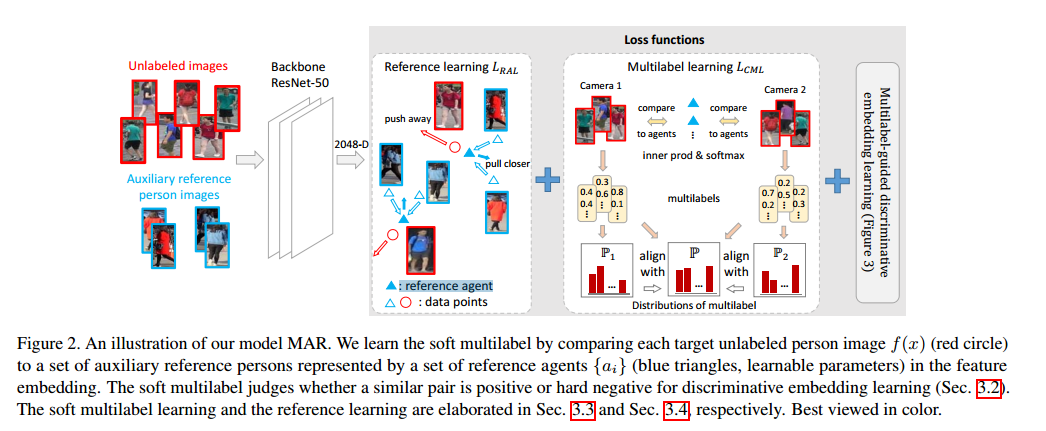
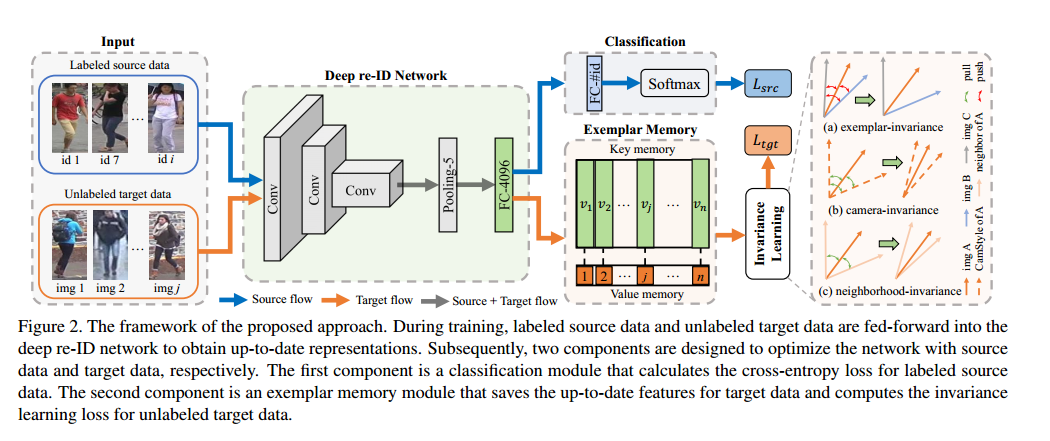
通过对比网络结构图可以看出,几种方法各有不同。
OIM(单数据集): 以 id 为索引,更新方式:running average,存储的是特征
DIMN: 以 id 为索引,更新方式:running average,存储的是经过线性变化的特征
MAR: 以 agent 的形式存在,更新方式:reference agent learning,存储的是特征
ECN: 以 index 为索引,更新方式:running average,存储的是特征
3. memory 的使用
OIM:
DIMN:
memory 中的特征是另一张图片的特征,而不是这个公式中$x_i$的特征。
MAR:
ECN: